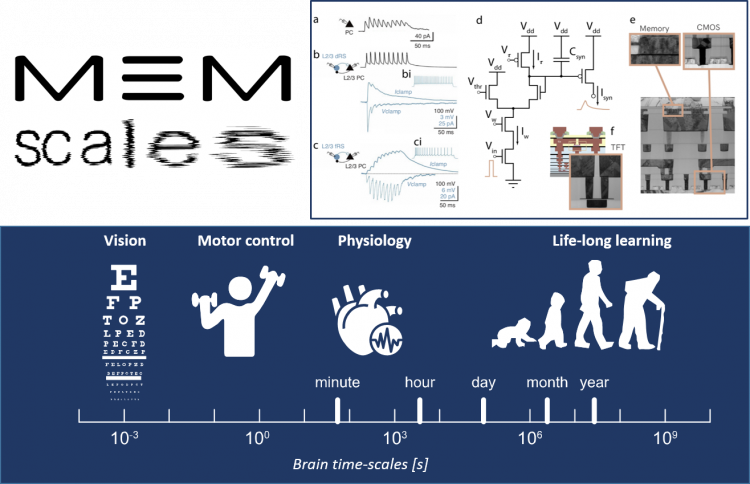
Neural processing in the nervous system occurs naturally over multiple time scales ranging from milliseconds (axonal transmission) to seconds (spoken phrases) and much longer intervals (motor learning).
In MeM-Scales we aim at building a novel class of neuromorphic computing systems that reproduce multi-timescale processing of biological neural systems, for building a novel class of neuromorphic computing systems that can process efficiently real-world sensory signals and natural time-series data in real-time (e.g. for low-power and always-on IoT and edge-computing applications that do not need to connect to the cloud), and to demonstrate this with a practical laboratory prototype.
The project consortium is broadly interdisciplinary, joining 9 international groups (Research centers, Universities and Industry) from material science through microchip design & manufacturing to computational neuroscience and machine learning.
Coordinator: CEA-Leti, France
Participants: IMEC, IMEC-NL, IBM, CNR-IMM, Consejo superior de Investigaciones Científicas (CSIC), University of Zurich, Univ. Groningen, aiCTX AG
Objectives. Our scientific and technological objectives can be summarized as follows:
- To study the theory, and develop algorithmic and architectural innovations for realizing adaptive and robust multi-timescale neural processing on mixed-signal analog/digital neuromorphic processors comprising both volatile and non-volatile memory devices to implement the synaptic circuits and TFT-based neurons.
- To develop novel hardware technologies that support on-chip learning with multiple time constants, both for synapses (volatile memory option combined with non-volatile memory, Electrochemical metallization, vacancy-type oxide-based memories, and Phase Change Memory), and neurons (TFT option exploration, plus integration with other devices).
- To study and develop an ultra-low-power, scalable and highly configurable neuromorphic computing processor capable of online, life-long learning for personalized neural learning and adaptation algorithms.
- To validate and demonstrate the project developments on realistic fully personalized edge application cases (by both simulation and board prototyping).
CNR Principal Investigator: Sabina Spiga
Involved Key Personnel: Stefano Brivio
Post Docs: Mrinmoy Dutta
Publications:
- Stefano Brivio, Denys R. B. Ly, Elisa Vianello and Sabina Spiga, Non-linear Memristive Synaptic Dynamics for Efficient Unsupervised Learning in Spiking Neural Networks, Frontiers in Neuroscience 15, 580909 (2021); https://doi.org/10.3389/fnins.2021.580909
W